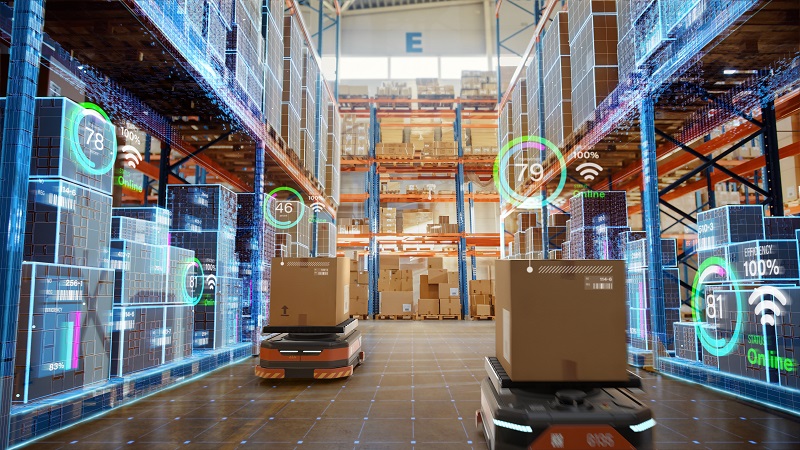
Data Overload: Could Even More Data Be A Good Thing?
We are awash with data. Digital technologies of all kinds are generating and capturing data at ever-increasing speed and volumes. But is this a good thing? Warehouse operators large and small have long realised that there is value in their data. Artificial intelligence (AI) and business intelligence (BI) tools are helping them release it but are themselves generating more data. If data is useful, could there be an argument to produce more of it to create opportunities for greater efficiencies and innovation? Cold Chain Federation member Principal Logistics Technologies ask the question.
The total amount of data created, captured, copied, and consumed globally reached around 64 zettabytes in 2020 and is predicted to grow to more than 180 zettabytes by the end of 2025. To put this into context, one zettabyte is 2 to the power of 70 bytes and it would take around one billion terabyte or one trillion gigabyte hard drives to store. By comparison a typical PC or laptop hard drive is around half a gigabyte. Headline figures like these are rarely broken down into industry sectors such as the supply chain but there is no doubt that applications such as warehouse management software (WMS) and enterprise resource planning (ERP) are generating and processing vast amounts of data globally.
The amount of data generated by such systems is going to increase. The global warehouse management systems market size was worth around $2.79bn in 2021 and is estimated to grow to about $7.52bn by 2028, according to one report. A separate report, covering a slightly different timeframe, suggests the warehouse management system market will grow from $3.2bn in 2023 to $9.9bn by 2030 . Either way this represents significant annual growth. And more systems will inevitably generate more data but individual installations and applications will also create more as they become increasingly sophisticated and interconnected with other systems including automation equipment.
- WMS market to grow from approximately $2.79bn in 2021 to up to $9.9bn by 2030
- WMS market to grow at compound annual growth rate of over 15% over next seven years
- Total global data produced predicted to treble by end of 2025
WMS generally captures and processes two types of data. The first, and the basis of all WMS, is broadly data about real-world operations and the characteristics of the items being handled. This might include an item’s identity and physical location as well as its weight and dimensions or other attributes chosen by stakeholders. The second type of data is created or processed as the item passes through the supply chain or warehouse. This can include where it came from, who delivered it, the date and time it arrived, the number of times it was handled between various parts of facility, whether or not it needs to be processed or prepared for delivery to its next destination, when and where it was packed, and by whom, and so on. These are probably the areas where warehouses are generating the bulk of new information.
All of this data is useful, essential even, for maintaining routine operations in the warehouse. Operators and developers realised years ago that this information was also valuable. WMS and other applications evolved to include analytical tools to identify patterns and trends and so on that release this value, for example by enabling process and performance improvements or supporting innovations and new ways of working. Analytics plays a pivotal role in running a more successful business, and new capabilities exist within today’s WMS to deliver a wide range of performance analytics and reporting that highlight how successful or unsuccessful your team is at delivering goods to your customers.
What is the significance of this? If data analytics can release value and enable innovation, it follows that the businesses that do this the best have the potential to be the most successful. But this could present an intriguing opportunity. If warehouses are generating more and more information about everyday operations, they have more that can provide insights and add value to their operations. As this type of information becomes easier (and less expensive) to capture, process, and store might it not be a good idea to deliberately capture more data and more often to generate and release even more value?
This is the concept of granularity: the more frequently you capture data about an item in the warehouse, the more you know about it. Tracking an item with high granularity as it moves through a warehouse, for example, can help reveal behaviour that might not otherwise be easily identifiable. As an illustration, imagine a WMS that instructs a pallet be handled to a specific aisle P&D station for placement into a racking location. In a traditional operation, the completion on this task would be seen as a “success” – a good thing – with little need for further thought.
But recording more information about the various stages will enable better and more useful analysis. Knowing how long each step took or how much time an item spent moving or was stationary, for example, could reveal whether any delays or bottlenecks occurred. Further analysis might show whether these had impacts elsewhere, for example by locking out the P&D space or delaying a lift truck movement and so on. That information could be used to introduce improvements to the process, such as prioritising specific actions over others or configuring the WMS to minimise the number of handling movements and distances and so on. This type of insight is useful in a conventional warehouse. But it could be even more critical when automation is involved because operations are faster and small changes have the potential to create big performance improvements.
Some of this analysis is already within the capabilities of WMS applications. Those with AI and BI capabilities (or the ability to integrate with specialist third party applications) go further by enabling even greater levels of insight. The challenge ahead may be to align data collection with required outcomes: collecting too little will lead to missed opportunities for improvement but collecting too much will be wasteful and complex with no additional insight or benefit.
Benefits of having more data to analyse:
- Improved decision-making
- Better customer service
- Increased efficiency
- Enhanced security
- Improved forecasting
Negative aspects of having too much data:
- Data overload
- Increased risk of data breaches (more data sources could mean more potential weak spots)
- Increased costs
- Difficulty in managing data quality and data privacy
New and emerging technologies such as AI and BI will change the data and business landscape in the next five years by:
- Increasing efficiency and productivity
- Improving decision-making processes
- Enhancing customer experience
- Reducing costs
- Providing better insights into customer behaviour
In addition to these benefits, having more data generated by automation of business applications such as warehouse management systems can lead to:
- Improved inventory management: With more data available, businesses can better track inventory levels and make more informed decisions about when to order new stock.
- Better supply chain management: By analysing data from across the supply chain, businesses can identify areas where they can improve efficiency and reduce costs.
- Improved customer service: By analysing customer data, businesses can gain insights into what customers want and need, allowing them to provide better service.
- Improved safety: By analysing safety data, businesses can identify areas where they need to improve safety procedures.
On the other hand, there are also negative aspects of having too much data generated by automation of business applications such as warehouse management systems:
- Data overload: With so much data available, it can be difficult for businesses to know what information is relevant and what is not.
- Increased risk of data breaches: With more data being generated, there is an increased risk of cyber attacks and data breaches.
- Increased costs: Collecting and analysing large amounts of data can be expensive.
- Difficulty in managing data quality: With so much data being generated, it can be difficult for businesses to ensure that the quality of the data is high.
- Difficulty in managing data privacy: With so much data being generated, it can be difficult for businesses to ensure that they are complying with privacy regulations.
New technologies such as AI and BI are changing the way that businesses operate. By generating more data through automation of applications such as WMS, businesses can gain valuable insights into their operations. However, there are also negative aspects of having too much data. As we move forward, it will be important for businesses to find ways to manage this data effectively while still reaping the benefits that it provides.
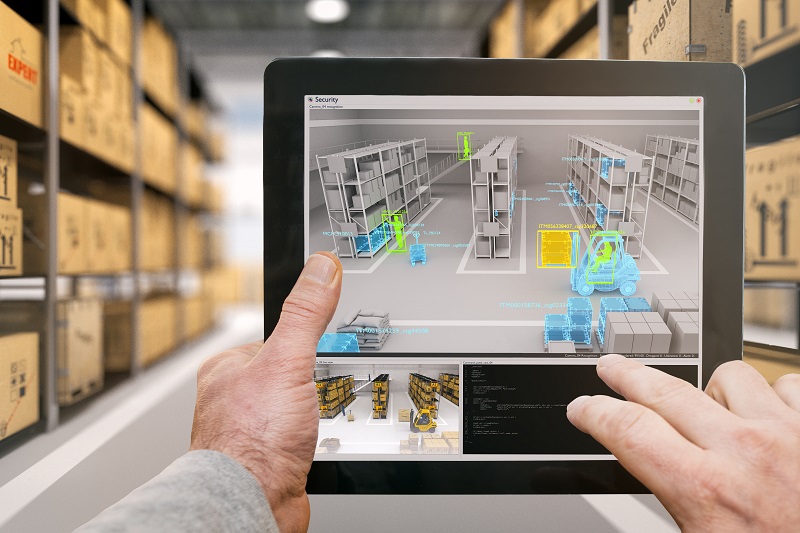
Comments are closed.